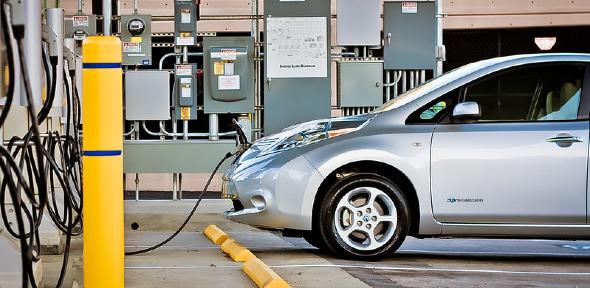
Researchers have developed a machine learning algorithm that could help reduce charging times and prolong battery life in electric vehicles by predicting how different driving patterns affect battery performance, improving safety and reliability.
The researchers, from the University of Cambridge, say their algorithm could help drivers, manufacturers and businesses get the most out of the batteries that power electric vehicles by suggesting routes and driving patterns that minimise battery degradation and charging times.
"This method could unlock value in so many parts of the supply chain, whether you’re a manufacturer, an end user, or a recycler, because it allows us to capture the health of the battery beyond a single number," Dr Alpha Lee, Yusuf Hamied Department of Chemistry
The team developed a non-invasive way to probe batteries and get a holistic view of battery health. These results were then fed into a machine learning algorithm that can predict how different driving patterns will affect the future health of the battery. If developed commercially, the algorithm could be used to recommend routes that get drivers from point to point in the shortest time without degrading the battery, for example, or recommend the fastest way to charge the battery without causing it to degrade.
The health of a battery, whether it’s in a smartphone or a car, is far more complex than a single number on a screen. “Battery health, like human health, is a multi-dimensional thing, and it can degrade in lots of different ways,” said first author Penelope Jones, from Cambridge’s Cavendish Laboratory. “Most methods of monitoring battery health assume that a battery is always used in the same way. But that’s not how we use batteries in real life. If I’m streaming a TV show on my phone, it’s going to run down the battery a whole lot faster than if I’m using it for messaging. It’s the same with electric cars – how you drive will affect how the battery degrades.”
The researchers developed a non-invasive probe that sends high-dimensional electrical pulses into a battery and measures the response, providing a series of ‘biomarkers’ of battery health. This method is gentle on the battery and doesn’t cause it to degrade any further.
The electrical signals from the battery were converted into a description of the battery’s state, which was fed into a machine learning algorithm. The algorithm was able to predict how the battery would respond in the next charge-discharge cycle, depending on how quickly the battery was charged and how fast the car would be going the next time it was on the road. Tests with 88 commercial batteries showed that the algorithm did not require any information about previous usage of the battery to make an accurate prediction.
Image credit: Derek Bruff