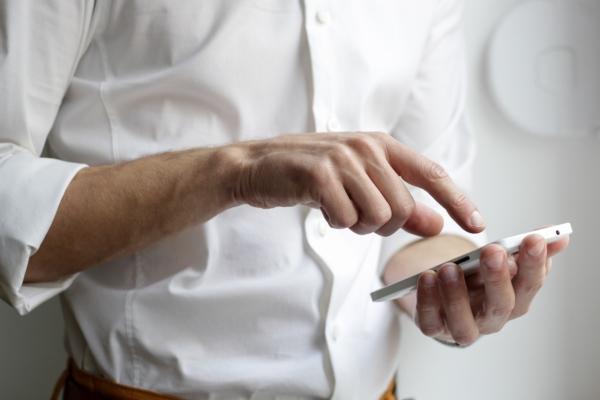
Researchers have designed a machine learning method that can predict battery health with 10x higher accuracy than current industry standard, which could aid in the development of safer and more reliable batteries for electric vehicles and consumer electronics.
The novel method monitors way to monitor batteries by sending electrical pulses into them and measuring the response. The measurements are then processed by a machine learning algorithm to predict the battery’s health and useful lifespan. Their method is non-invasive and is a simple add-on to any existing battery system.
Predicting the state of health and the remaining useful lifespan of lithium-ion batteries is one of the big problems limiting widespread adoption of electric vehicles: it’s also a familiar annoyance to mobile phone users. Over time, battery performance degrades via a complex network of subtle chemical processes. Individually, each of these processes doesn’t have much of an effect on battery performance, but collectively they can severely shorten a battery’s performance and lifespan.
A machine learning model is used to discover specific features in the electrical response that are the tell-tale sign of battery aging. The researchers performed over 20,000 experimental measurements to train the model, the largest dataset of its kind. Importantly, the model learns how to distinguish important signals from irrelevant noise. Their method is non-invasive and is a simple add-on to any existing battery systems.
"Safety and reliability are the most important design criteria as we develop batteries that can pack a lot of energy in a small space. By improving the software that monitors charging and discharging, and using data-driven software to control the charging process, I believe we can power a big improvement in battery performance."
Click here for the full University of Cambridge article.
Click here for the Nature Communications publication - "Identifying degradation patterns of lithium ion batteries from impedance spectroscopy using machine learning"
Image credit: Nordwood Themes via Unsplash.